Spotlight: Ben Grassian January 5, 2024
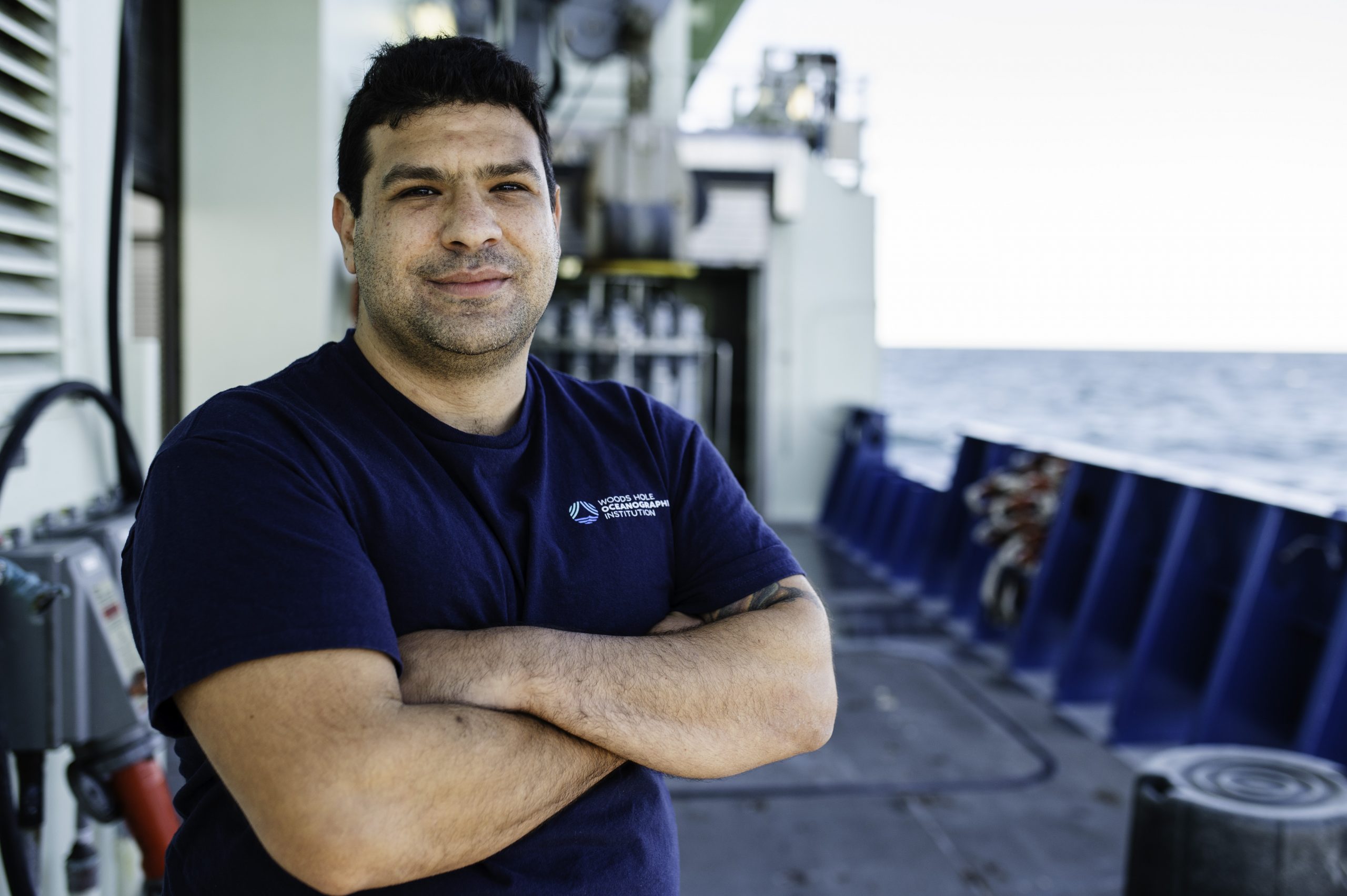
As a postdoctoral fellow with WHOI’s Twilight Zone project, Ben Grassian wears plenty of hats. In the interview below, learn how Grassian splits his time between biology and acoustical oceanography, while developing complex artificial intelligence systems that could help to improve both fields.
How did you get your start working with AI?
I started out in the biological sciences, but in grad school, I worked in a marine robotics lab where I helped to develop technology aboard new deep-sea vehicles. That really opened my eyes. I was really interested in life in the water column, since the midwaters of the ocean make up a huge area of the Earth that is largely unexplored and inhospitable to humans—but by using AI and machine learning, we can now collect these in-situ measurements of marine life from multiple instruments, and analyze them at a rate that just wasn’t feasible in the past. That is pretty unique, because it gives us a way to comb through massive datasets, find connections between them, and gain meaningful insights into what is down there and how those animals are living. Getting a complete picture of midwater habitats requires the use of complimentary sampling techniques, like imaging, acoustics, and net tows, which is why I have sought out these multidisciplinary sampling approaches.
What drew you to the OTZ project?
I really liked that the OTZ project was focused on using a diverse set of complementary measurements to get a full picture of the twilight zone ecosystem. If you only use acoustics, you can make these really broad observations of the water column, but figuring out individual species or families is a really tricky problem. Likewise, if you only use image data, you can get a much more specific measurement of individual animals and their identities, but you don’t get a big-picture view of a region instantaneously. That’s why, as a postdoc, I’m splitting my time between the labs of [acoustical oceanographer] Andone Lavery and [biologist] Heidi Sosik—we’re creating AI tools to help link those fields together for a more comprehensive look at the twilight zone residents.
How are you working to do that?
The Ocean Twilight Zone project has this really great shadowgraph imaging system called ISIIS, which collects 15 frames per second, and it’s often running for hours at a time. So you wind up with a huge amount of image data that would be kind of left on the table without machine learning tools. A human user can't feasibly go through all those images and do it themselves, but AI tools can do it in a relatively short amount of time.
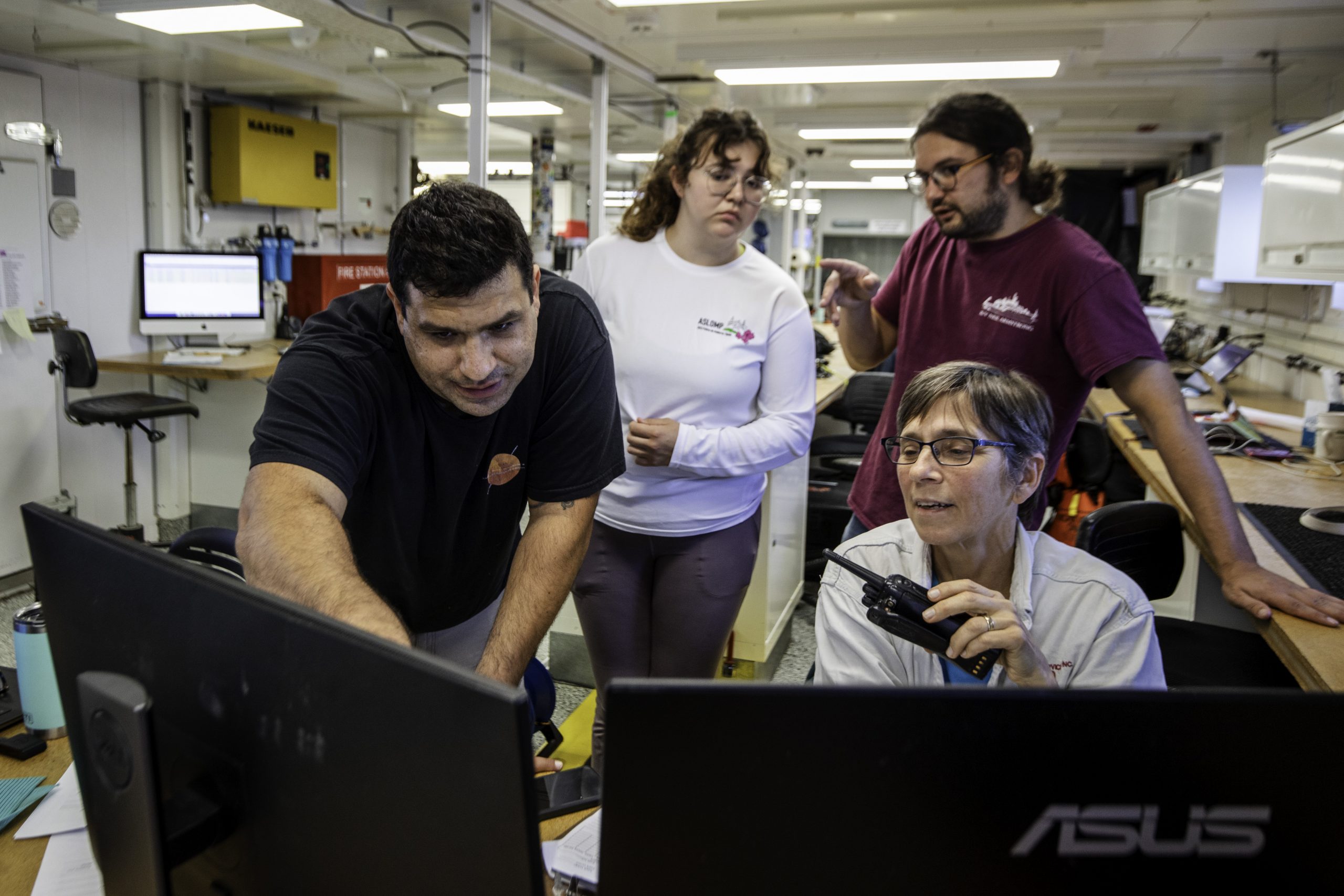
What are some of the big questions that your work could help OTZ scientists answer?
You could apply this research to a lot of different questions. What are the animal compositions in the twilight zone? What are their habits and their distributions within the local environment? By using AI techniques on imagery we get from our instruments, we can gain valuable information about which species are in a specific location, and then compare that to the acoustic data we’ve got. Doing these comparisons between the animals resolved optically and acoustically will allow us to find more precise ways to classify acoustic data and find patterns within it.
What’s the most difficult part of using AI to analyze all that data?
I’d say training it correctly. For a human, it's so simple to say, ok, this picture shows an animal with six legs—but for a computer to tell that, it has to mathematically know what a leg even is, and how it can identify it in a digital image. Using machine learning, a computer can kind of figure that part out on its own, but you have to teach it first by training it on representative examples. That involves curating a huge set of images, often thousands of them, that already have the variety of animals of interest correctly identified—and then you feed those training sets to construct models that can be used to infer animal identities in unseen images. Artificial intelligence isn’t perfect; it still needs a lot of human input. Even if it seems like a magic black box, you actually have to babysit it statistically to know how well it’s working.