Spotlight: Emma Cotter February 25, 2021
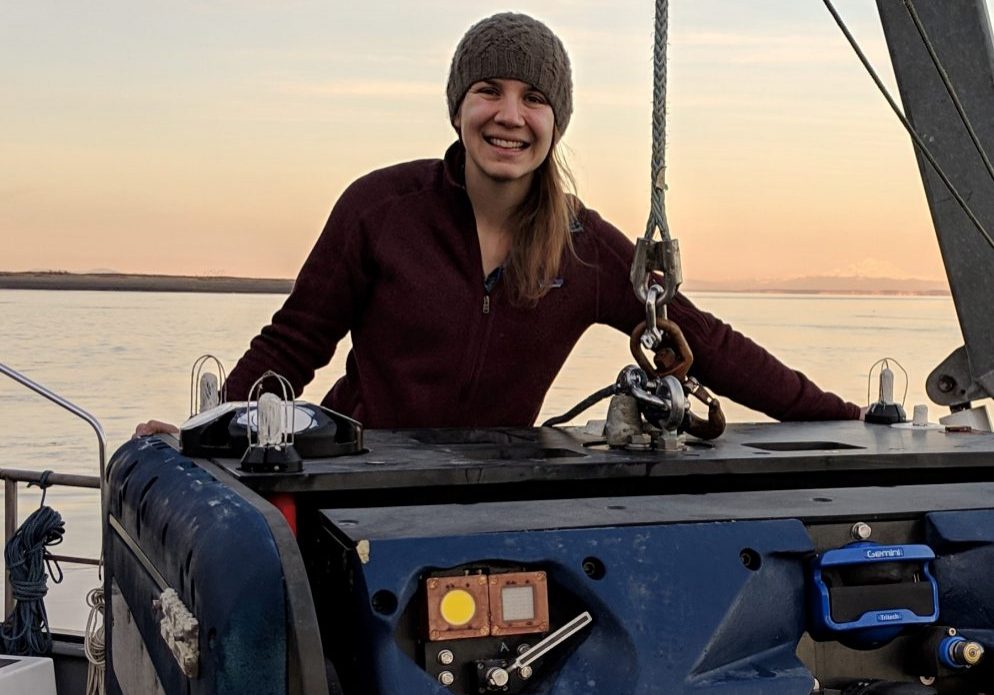
As robots and instruments used in ocean research collect more and more data, analyzing it all will be a real challenge. That’s where WHOI’s Emma Cotter comes in—she specializes in making sense of massive data sets, and has created machine learning algorithms that can sort through them in just a fraction of the time of humans. In this interview, learn about Cotter’s passion for machine learning, her experience working on new instruments for WHOI, and much more.
You’ve focused pretty extensively on analyzing acoustic data in your work—what drew you to that?
That was one thing I really wanted to learn in my postdoctoral position. I had been working on machine learning for acoustic instruments, but didn’t have a very strong base in the underlying physics of acoustics. I really wanted to learn more about that side of the problem, and develop machine learning that could incorporate it. I feel like I've been able to learn a lot about that—It sort of put me outside of my comfort zone, which is a good way to figure out how to address new problems.
The real advantage of working with acoustics, as opposed to camera-based sensors, is that we can measure things in the deep ocean from a relatively long distance away, and we don't need any light to do that. It gives us a sense of what’s happening right now in the twilight zone without needing to sneak right up on an organism. Another advantage is that you're not just getting information about one point far away from you: acoustics let you survey a relatively large area in one shot, and give you much broader spatial measurements than you're able to get with most underwater cameras. The challenge really lies in interpreting the data, and figuring out what you’re looking at. That’s pretty complicated. We're not able to get the same level of classification that we’d be able to get with a camera—we might be able to tell how many fish are there, or roughly what size they are, but we probably won’t be able to tell which species they are from the acoustics alone. If we combine data from a bunch of different sources, though, we can start to get a better sense of what organisms exist in the twilight zone.
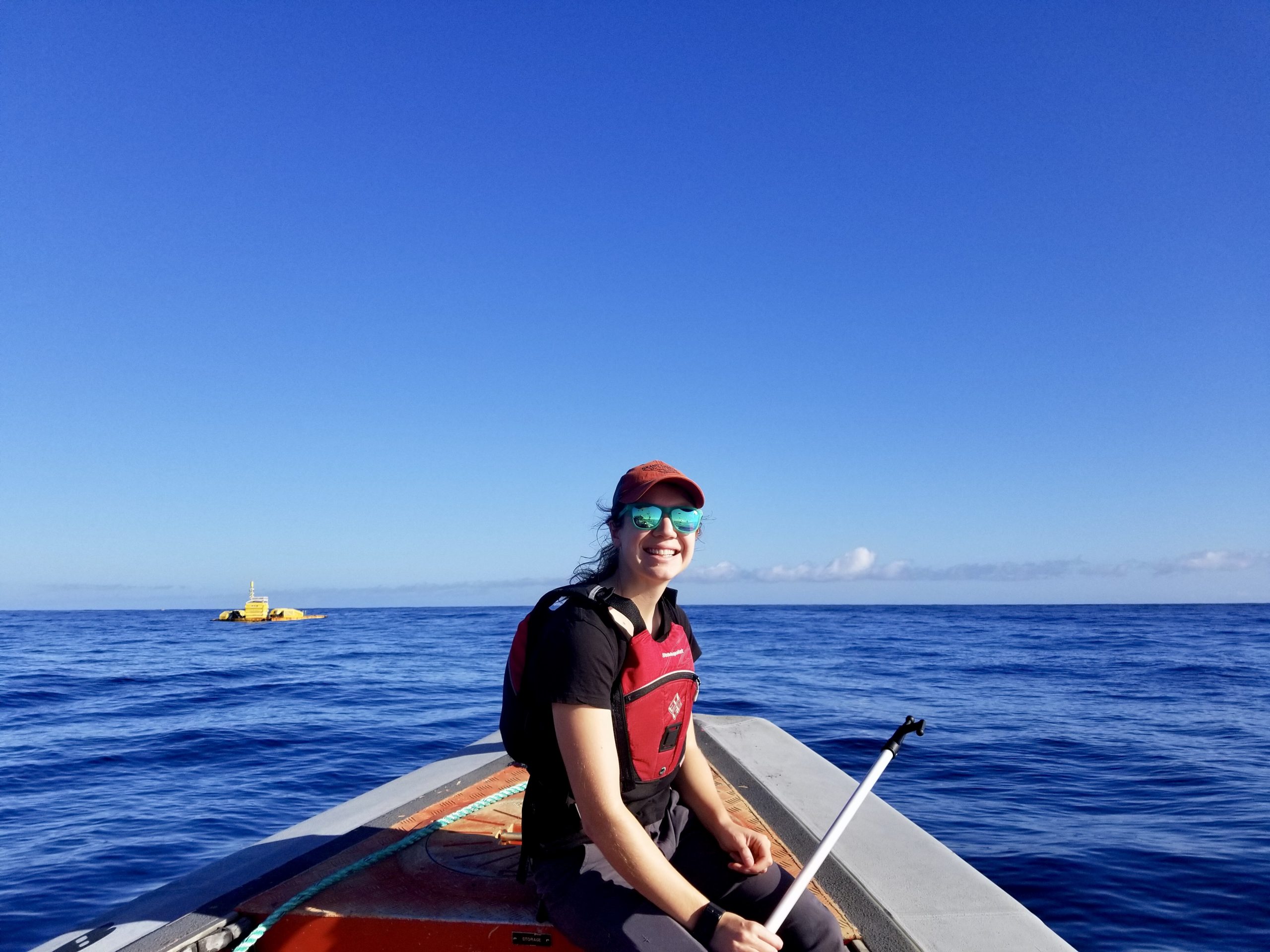
How did you first get interested in studying the oceans?
I grew up in Rhode Island, so I always loved the ocean. When I was applying to grad school, I came across a group at the University of Washington that was studying marine renewable energy, like generating electricity from tidal turbines and wave energy—and it just kind of clicked that this was something I wanted to do. My PhD focused on environmental monitoring technologies for those energy devices. Just like we need to consider migrating birds when designing wind farms, we need to understand how marine energy devices interact with the environment. Monitoring those animals under water for long periods of time can be challenging, though, so I was working on a system that used cameras, and hydrophones and multi beam sonar in one package to determine what animals were nearby. My main goal was to combine all those sensing platforms with real-time machine learning, which can then train the system to detect if seals or other marine mammals are in the area.
How does that experience translate into the work you’re doing at WHOI?
At Woods Hole, I work with Andone Lavery on the Deep-See platform, which is very similar in a way to those monitoring systems. It has a whole bunch of instruments that work together —cameras, multibeam sonar, and so on—and they’re all producing a ton of data. So how do we handle that data, and break it down so we can answer the environmental questions that we have? That's sort of where I come in.
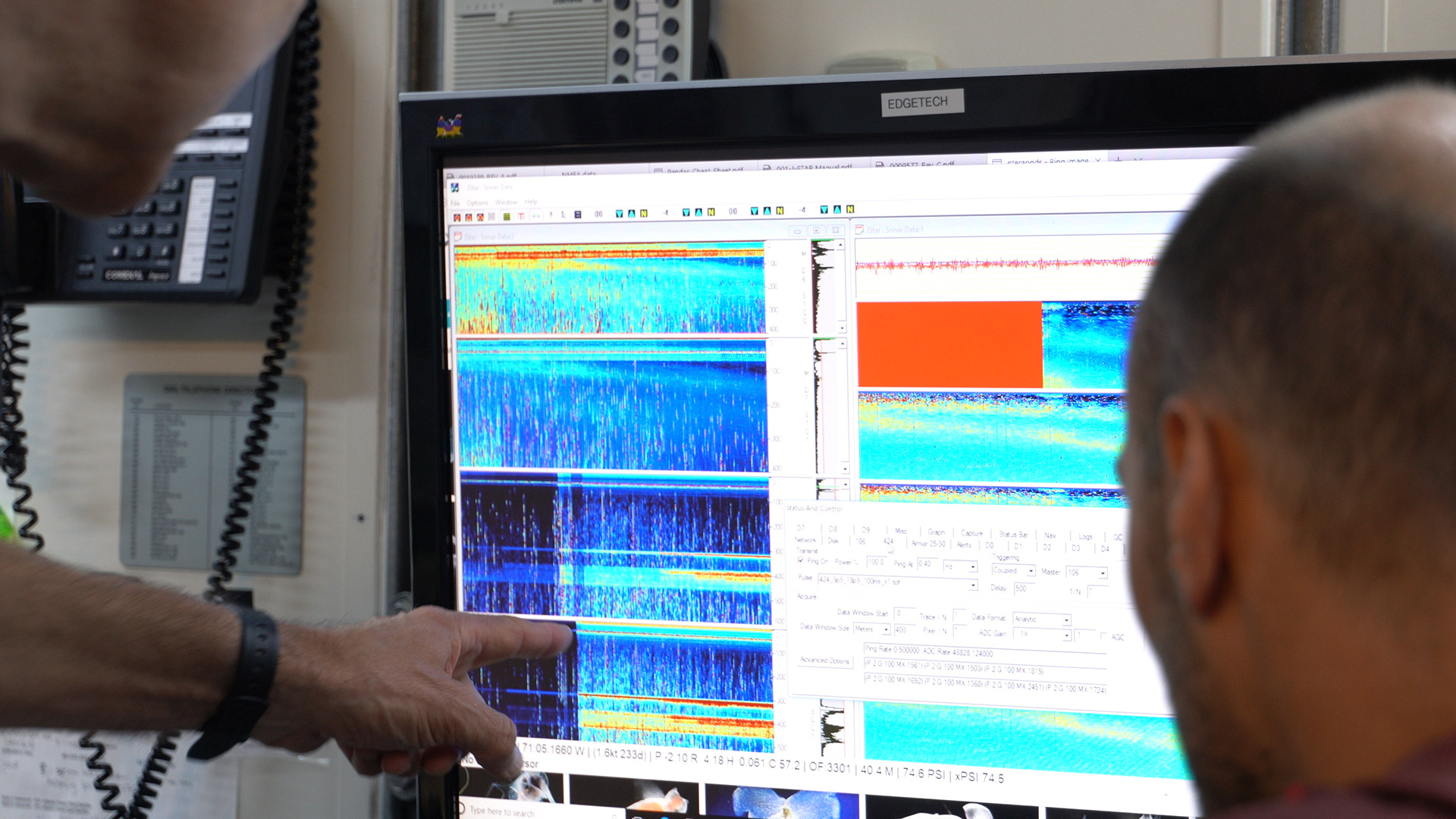
What other ways are you using machine learning to study the twilight zone?
The real advantage of working with acoustics, as opposed to camera-based sensors, is that we can measure things in the deep ocean from a relatively long distance away, and we don't need any light to do that. It gives us a sense of what’s happening right now in the twilight zone without needing to sneak right up on an organism. Another advantage is that you're not just getting information about one point far away from you: acoustics let you survey a relatively large area in one shot, and give you much broader spatial measurements than you're able to get with most underwater cameras. The challenge really lies in interpreting the data, and figuring out what you’re looking at. That’s pretty complicated. We're not able to get the same level of classification that we’d be able to get with a camera—we might be able to tell how many fish are there, or roughly what size they are, but we probably won’t be able to tell which species they are from the acoustics alone. If we combine data from a bunch of different sources, though, we can start to get a better sense of what organisms exist in the twilight zone.
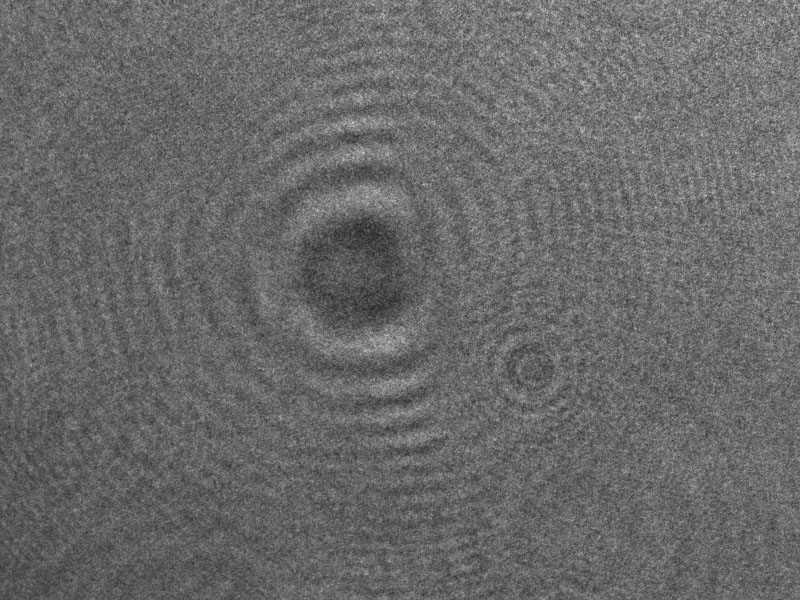
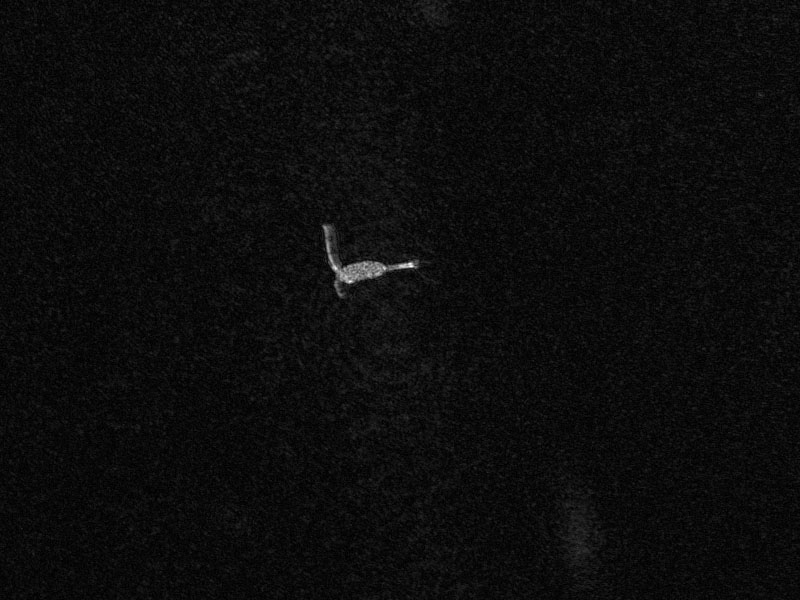
What are some of the biggest surprises you’ve encountered while studying the ocean twilight zone?
Well, since I’m new to studying the deep ocean and new to using broadband acoustics and holographic cameras, there are lots of surprises every day. But personally, it’s been incredible to see how much life and biodiversity there is in the deep ocean. It’s also been a little bit surprising to learn just how complicated it is to study. We need all of these different mechanisms and technologies to start wrapping our heads around it. I don't think any one instrument is going to give us the answer that we need. It's a really, really complex and interesting problem.
What do you love most about your work? What keeps you going?
There's so much that we need to know about the oceans. Understanding how it fits into human life and how it fits into huge questions, like how climate is changing, is incredibly important. I really enjoy working with big, confusing datasets that we collect in the ocean, and then figuring out how to come up with efficient and robust tools to answer those scientific questions. That's really exciting. Being able to handle huge amounts of data is very important right now, especially since we’re getting better and better at collecting it in the deep ocean. What drives me is figuring out how we can get the most out of those rich and complicated datasets.